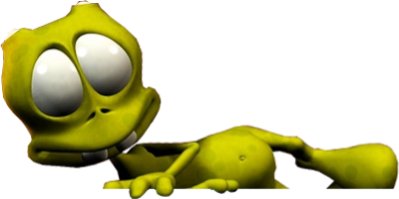
EAN: 9783031010279
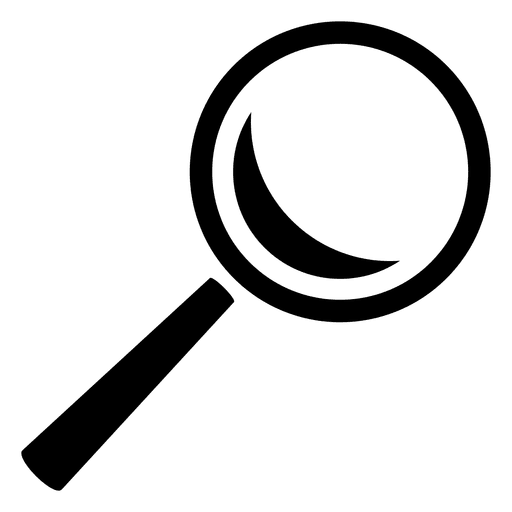
Bilder-Quelle: discount24.de - Sport-Freizeit
Learning to rank refers to machine learning techniques for training a model in a ranking task. Learning to rank is useful for many applications in information retrieval natural language processing and data mining. Intensive studies have been conducted on its problems recently and significant progress has been made. This lecture gives an introduction to the area including the fundamental problems major approaches theories applications and future work. The author begins by showing that various ranking problems in information retrieval and natural language processing can be formalized as two basic ranking tasks namely ranking creation (or simply ranking) and ranking aggregation. In ranking creation given a request one wants to generate a ranking list of offerings based on the features derived from the request and the offerings. In ranking aggregation given a request as well as a number of ranking lists of offerings one wants to generate a new ranking list of the offerings. Ranking creation (or ranking) is the major problem in learning to rank. It is usually formalized as a supervised learning task. The author gives detailed explanations on learning for ranking creation and ranking aggregation including training and testing evaluation feature creation and major approaches. Many methods have been proposed for ranking creation. The methods can be categorized as the pointwise pairwise and listwise approaches according to the loss functions they employ. They can also be categorized according to the techniques they employ such as the SVM based Boosting based and Neural Network based approaches. The author also introduces some popular learning to rank methods in details. These include: PRank OC SVM McRank Ranking SVM IR SVM GBRank RankNet ListNet & ListMLE AdaRank SVM MAP SoftRank LambdaRank LambdaMART Borda Count Markov Chain and CRanking. The author explains several example applications of learning to rank including web search collaborative filtering definition search keyphrase extraction query dependent summarization and re-ranking in machine translation. A formulation of learning for ranking creation is given in the statistical learning framework. Ongoing and future research directions for learning to rank are also discussed. Table of Contents: Learning to Rank Learning for Ranking Creation Learning for Ranking Aggregation Methods of Learning to Rank Applications of Learning to Rank Theory of Learning to Rank Ongoing and Future Work
Produktinformationen zuletzt aktualisiert am
17.06.2025 um 01:34 Uhr
17.06.2025 um 01:34 Uhr
Hersteller
-
EAN
9783031010279
MPN
-
ASIN
3031010272
Produktgruppe
-
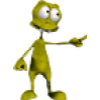
Produktzustand:
Verfügbarkeit:
Versandkosten:
Sonderpreis:

Sie sind Shopbetreiber? Listen Sie ganz einfach Ihre Produkte hier bei uns im Portal >>>
Letzte EAN Aktualisierungen:
9783031796289 - Synthesis Lectures on Mechanical Engineering Nat...9783031007576 - Synthesis Lectures on Data Management Full-Text ...
9783031795039 - Synthesis Lectures on Sustainable Development Oi...
9783031010576 - Synthesis Lectures on Human-Centered Informatics ...
9783031798788 - Synthesis Lectures on Digital Circuits & Systems ...
9783031798023 - Synthesis Lectures on Digital Circuits & Systems ...
9783031008757 - Synthesis Lectures on Distributed Computing Theory...
9783031005534 - Synthesis Lectures on Communications Partial Upd...
9783031012778 - Synthesis Lectures on Mathematics & Statistics A...
kürzlich hinzugefügt:
9783031795039 - Synthesis Lectures on Sustainable Development Oi...9783031010576 - Synthesis Lectures on Human-Centered Informatics ...
9783031798023 - Synthesis Lectures on Digital Circuits & Systems ...
9783031796289 - Synthesis Lectures on Mechanical Engineering Nat...
9783031007576 - Synthesis Lectures on Data Management Full-Text ...
9783031008757 - Synthesis Lectures on Distributed Computing Theory...
9783031012778 - Synthesis Lectures on Mathematics & Statistics A...
9783031798788 - Synthesis Lectures on Digital Circuits & Systems ...
9783031005534 - Synthesis Lectures on Communications Partial Upd...